NUS-SKKU FinTech Seminars
The NUS–SKKU FinTech Seminar Series is a collaborative effort by the Asian Institute of Digital Finance (AIDF) at NUS and Department of FinTech at SKKU. The series aims to foster interdisciplinary discussions on the FinTech research that are relevant to the scientific community and the industry.
The monthly seminars when the two universities are in session will be conducted in a hybrid format. The speaker delivers the talk onsite (either in Singapore or Seoul) or online, and the participants can choose to join a seminar onsite or online. The seminar time is Friday (10am-11.30am Singapore; 11am-12.30pm Seoul), which may be moved on a need basis to 2pm-3.30pm Singapore or 3pm–4.30pm Seoul to accommodate the online speaker. Seminars are free but require registrations.
Join our mailing list to keep up to date on our FinTech Seminars
2023 - Past FinTech Seminars
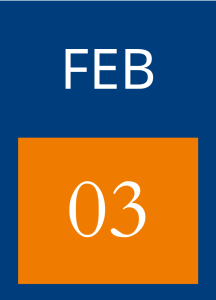
A Data-driven and Interpretable Topic Model for Management Disclosures
Matthias Fengler
University of St.Gallen
Abstract
The paper investigates the topics discussed in the Management Discussion and Analysis (MD&A) section of 10-K filings from 1994.01 until 2018.12. In our modeling approach, we elicit the MD&A topics by clustering words around a set of anchor words that broadly define a potential topic. Given the topics, we extract two hidden loading series from the MD&As – a measure of topic prevalence and a measure of topic sentiment. The results are four-fold. First, we find that the topics we extract are intelligible and distinctive but potentially multi-modal, which may explain why classical topic models applied to 10-K filings often lack interpretability. Second, the topic loadings tend to follow local trends which often can be explained historically. Third, our measures of topic sentiment exhibit that sentiment does not affect topics uniformly but in rather topic-specific nuanced ways. Finally, we find that MD&A topic compilation exhibits systematic variation across firm characteristics.
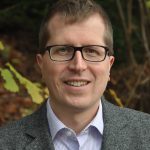
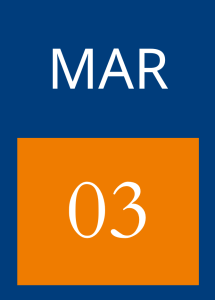
Seoul National University
Abstract
Decentralized autonomous organizations (DAOs) operate based on a set of decisionmaking rules encoded into smart contracts utilizing blockchain technology. They lack a central point of authority in contrast to traditional corporations. In our paper, we develop a theoretical model of DAO governance featuring strategic token trading and a token-based voting system to investigate potential conflicts of interest between a large participant (“whale”) and many small participants. Our results show that ownership concentration is negatively related to platform growth, but platform size, token illiquidity, and long-term incentives can mitigate the negative effects. We confirm these predictions using novel voting data on more than 200 DAO projects from July 2020 to July 2022.
Research Paper
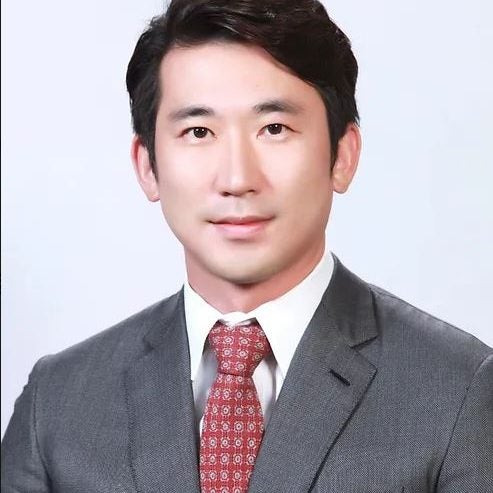
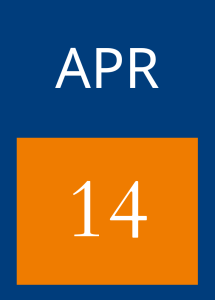
Reinforcement Learning for Quantitative Decision Making in Financial Markets
Bo An
Nanyang Technological University
Abstract
In the last decade, we have witnessed a significant development of AI-powered quantitative trading (QT), due to its instant and accurate order execution, and capability of analyzing and processing large amount of data related to the financial market. Traditional AI-powered QT methods discover trading opportunities based on either heuristic rules or financial prediction. However, due to the high volatility and noisy nature of financial market, their performance is not stable and highly reply on the market condition. Recently, reinforcement learning (RL) becomes an appealing approach for QT tasks owing to its stellar performance on solving complex decision-making problems. This talk will discuss some research progress in RL for QT and future directions. This talk will also discuss some other RL’s applications in games, e-commerce, and urban planning.
Research Papers
- Shuo Sun, Molei Qin, Xinrun Wang, Bo An. PRUDEX-Compass: Towards systematic evaluation of reinforcement learning in financial markets. Transactions on Machine Learning Research (TMLR), accepted. [PDF]
- Shuo Sun, Rundong Wang, Bo An. Reinforcement learning for quantitative trading. ACM Transactions on Intelligent Systems and Technology, accepted. [PDF]
- Shuo Sun, Rundong Wang, Wanqi Xue, Xu He, Junlei Zhu, Jian Li, Bo An. DeepScalper: A risk-aware reinforcement learning framework to capture fleeting intraday trading opportunities. Proceedings of the 31st ACM International Conference on Information and Knowledge Management (CIKM'22), pp.1858-1867. [PDF]
- Rundong Wang, Hongxin Wei, Bo An, Zhouyan Feng, Jun Yao. Commission fee is not enough: A hierarchical reinforced framework for portfolio management. Proceedings of the 35th AAAI Conference on Artificial Intelligence (AAAI'21), pp.626-633. [PDF]
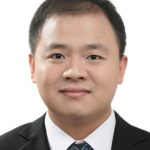
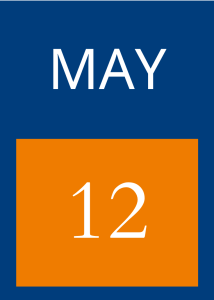
Predicting Bond Returns with Machine Learning: A Critical Appraisal of the Spanning Hypothesis
University of Illinois Urbana-Champaign
Abstract
The bond risk premia spanning hypothesis posits that the yield curve contains ample pricing information regarding bond risk premia, enabling the prediction of future bond returns solely based on the yield curve. In this study, we employ neural networks to investigate the predictive power of the yield curve on future bond returns and undertake a critical evaluation of the spanning hypothesis as well as previous research in this field.
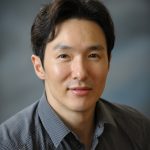
2023 - Past FinTech Seminars

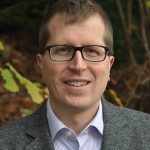
A Data-driven and Interpretable Topic Model for Management Disclosures
Matthias Fengler
University of St.Gallen
Abstract
The paper investigates the topics discussed in the Management Discussion and Analysis (MD&A) section of 10-K filings from 1994.01 until 2018.12. In our modeling approach, we elicit the MD&A topics by clustering words around a set of anchor words that broadly define a potential topic. Given the topics, we extract two hidden loading series from the MD&As – a measure of topic prevalence and a measure of topic sentiment. The results are four-fold. First, we find that the topics we extract are intelligible and distinctive but potentially multi-modal, which may explain why classical topic models applied to 10-K filings often lack interpretability. Second, the topic loadings tend to follow local trends which often can be explained historically. Third, our measures of topic sentiment exhibit that sentiment does not affect topics uniformly but in rather topic-specific nuanced ways. Finally, we find that MD&A topic compilation exhibits systematic variation across firm characteristics.

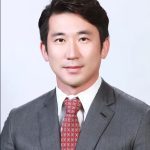
Seoul National University
Abstract
Decentralized autonomous organizations (DAOs) operate based on a set of decisionmaking rules encoded into smart contracts utilizing blockchain technology. They lack a central point of authority in contrast to traditional corporations. In our paper, we develop a theoretical model of DAO governance featuring strategic token trading and a token-based voting system to investigate potential conflicts of interest between a large participant (“whale”) and many small participants. Our results show that ownership concentration is negatively related to platform growth, but platform size, token illiquidity, and long-term incentives can mitigate the negative effects. We confirm these predictions using novel voting data on more than 200 DAO projects from July 2020 to July 2022.
Research Paper

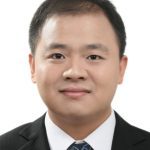
Reinforcement Learning for Quantitative Decision Making in Financial Markets
Bo An
Nanyang Technological University
Abstract
Decentralized autonomous organizations (DAOs) operate based on a set of decisionmaking rules encoded into smart contracts utilizing blockchain technology. They lack a central point of authority in contrast to traditional corporations. In our paper, we develop a theoretical model of DAO governance featuring strategic token trading and a token-based voting system to investigate potential conflicts of interest between a large participant (“whale”) and many small participants. Our results show that ownership concentration is negatively related to platform growth, but platform size, token illiquidity, and long-term incentives can mitigate the negative effects. We confirm these predictions using novel voting data on more than 200 DAO projects from July 2020 to July 2022.
Research Papers
- Shuo Sun, Molei Qin, Xinrun Wang, Bo An. PRUDEX-Compass: Towards systematic evaluation of reinforcement learning in financial markets. Transactions on Machine Learning Research (TMLR), accepted. [PDF]
- Shuo Sun, Rundong Wang, Bo An. Reinforcement learning for quantitative trading. ACM Transactions on Intelligent Systems and Technology, accepted. [PDF]
- Shuo Sun, Rundong Wang, Wanqi Xue, Xu He, Junlei Zhu, Jian Li, Bo An. DeepScalper: A risk-aware reinforcement learning framework to capture fleeting intraday trading opportunities. Proceedings of the 31st ACM International Conference on Information and Knowledge Management (CIKM'22), pp.1858-1867. [PDF]
- Rundong Wang, Hongxin Wei, Bo An, Zhouyan Feng, Jun Yao. Commission fee is not enough: A hierarchical reinforced framework for portfolio management. Proceedings of the 35th AAAI Conference on Artificial Intelligence (AAAI'21), pp.626-633. [PDF]

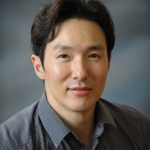
Predicting Bond Returns with Machine Learning: A Critical Appraisal of the Spanning Hypothesis
University of Illinois Urbana-Champaign
Abstract
The bond risk premia spanning hypothesis posits that the yield curve contains ample pricing information regarding bond risk premia, enabling the prediction of future bond returns solely based on the yield curve. In this study, we employ neural networks to investigate the predictive power of the yield curve on future bond returns and undertake a critical evaluation of the spanning hypothesis as well as previous research in this field.